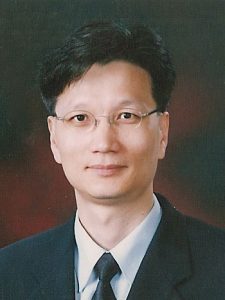 |
|
Dr. Sung-Bae Cho, Professor, Department of Computer Science, Yonsei University, Seoul, Korea.
Dr. Cho received the Ph.D. degree in computer science from KAIST (Korea Advanced Institute of Science and Technology), Korea, in 1993. He was an Invited Researcher of Human Information Processing Research Laboratories at Advanced Telecommunications Research (ATR) Institute, Japan from 1993 to 1995, and a Visiting Scholar at University of New South Wales, Australia in 1998. He was also a Visiting Professor at University of British Columbia, Canada from 2005 to 2006. Since 1995, he has been a Professor in Department of Computer Science, Yonsei University, Korea. Dr. Cho has been serving as an associate editor for several journals including IEEE Transactions on CI and AI on Games (2009-present) and IEEE Transactions on Fuzzy Systems (2013-present). He was also the chair of Games Technical Committee, IEEE CIS (2009-2010), and Student Games-based Competition Subcommittee, IEEE CIS (2011-2012). He is a member of Board of Government (BoG) of Asia Pacific Neural Networks Assembly (APNNA) (2011-present), and a member of three technical committees in IEEE CIS such as Emergent Technologies, Computational Finance and Economics, and Games. His research interests include hybrid intelligent systems, soft computing, evolutionary computation, neural networks, pattern recognition, intelligent man-machine interfaces, and games. He has published over 230 journal papers, and over 680 conference papers.
Abstract:
Title: Prospects and Challenges of Hybrid Deep Learning
Recently, deep learning opens another renaissance of artificial intelligence that is a long dream of human-beings. There are four representative models for deep learning, and two of them can be convolutional neural networks and recurrent neural networks. In this talk, I will give the general idea of both methods, and discuss about the prospects and challenges. Especially, to work out realistic problems, we need a hybrid architecture of several deep learning models. I will also present a generative model via an adversarial process gets a great attention due to the amazing demonstration of performance. It can simultaneously train a generative model to capture the data distribution, and discriminative model to estimate the probability that a sample came from the training data. In this talk, we present a new method of transfer-generative adversarial network (tGAN) with auto-encoders to detect anomaly in malicious software (malware) for computer security. Experiments with the malware dataset from the Kaggle Microsoft malware classification challenge (https://www.kaggle.com/c/malware-classification) show that the tGAN achieves 95.74% average classification accuracy which is higher than accuracy of other state-of-the-art methods and increases the learning stability. |